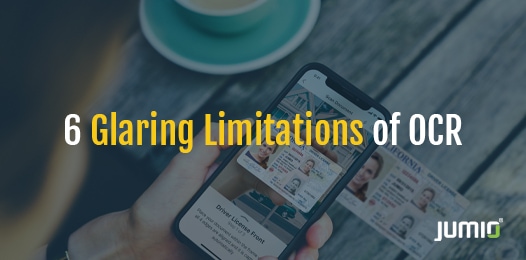
We’re starting to observe a new market phenomenon: the rise of DIY online identity verification and efforts by companies to cobble together OCR technology, facial recognition software and low-cost manual review teams.
Want to learn more now? Download Jumio’s eKYC in APAC Guide.
At its face, this approach makes some sense, but it’s important to understand how these technologies work and understand their inherent limitations.
Let’s start with optical character recognition (OCR), which can be used to extract important data from an ID document, such as a driver’s license or passport. This will generally include a person’s name, address, date of birth and ID number. The data extraction process is usually fast and reduces or removes the need for manual data input.
But, OCR is not without its challenges.
OCR was originally intended for reading black text against a white background often using a flatbed scanner — not for extracting key data fields from ID documents using small fonts and different colored backgrounds that may include holograms, watermarks and printing on glossy surfaces.
Here are six real-world limitations of OCR when applied to data extraction from pictures of ID documents:
1. Structuring the Data Involves More than Just OCR
When users take a picture of their ID document with their smartphone or webcam, multiple steps are required to extract and structure the information. The first step is to precisely recognize what kind of ID document is present. This enables the engine to properly structure the information read with the OCR, which means figuring out the first name, last name, date of birth and any other field of interest. Straight OCR without additional AI or technology specifically trained to recognize ID types will lack the requisite accuracy you need to fight fraud and deliver a good user experience.
2. OCR Must Combine with Image Rectification
When people take pictures of their ID documents using their smartphones or webcams, these images usually need to be de-skewed if the image was not aligned properly and reoriented so that the OCR technology can properly extract the data.
3. IDs with Colored Backgrounds Can Be Problematic for OCR
OCR often must take a color/grayscale photo and convert it to plain black and white to reduce blurred text and better separate black and white text from its background.
4. Glare and Blur Can Cause Mistakes
What happens if there is glare or the user moves a bit while the picture of their ID is captured? When there is glare or blurriness in the ID image, the probability of data extraction mistakes is significantly higher.
5. Webcams are a Challenge for Traditional OCR
OCR poses another challenge for companies looking to offer an omnichannel experience by enabling customers to capture ID documents through a variety of channels. While the cameras embedded into most smartphones are of high quality and take high-resolution pictures, this is not the case for the webcams built into desktops and tablets. If a company lets their users verify their IDs via webcam, this will impact the quality and clarity of the ID picture which, in turn, challenges the ability of OCR to correctly extract the data.
6. OCR May Be Challenged by Some ID Subtypes
OCR is based, in part, on an extensive learning of the patterns that characterize a specific ID type, and this can make for a challenging learning task given the variety of ID subtypes (e.g. some printed in landscape, some in portrait mode). OCR is only fully usable if the data extracted is correctly structured and that requires the software to understand all the nuances and subtleties of different ID types around the globe.
These real-world limitations for extracting data from pictures of ID documents often go well beyond the original design of OCR. Unfortunately, OCR is usually not set up to address these circumstances which limits its ability as a standalone engine.
A group of researchers from the University of Wisconsin-Milwaukee proved the real-world limitations of OCR in a recent research paper that compared four popular OCR solutions: Google Docs OCR, Tesseract, ABBY Fine Reader and Transym. These OCR systems performed reasonably well when extracting characters from digital images under normal conditions with accuracy levels between 79 percent and 88 percent. But, when tested with blurred images and skewed images, the accuracy of these engines plummeted to between 28 percent and 62 percent. This demonstrates that even with leading “off the shelf” OCR solutions, data extraction accuracy is dependent on the quality of the input images.
It’s because of these inherent limitations that leading identity verification solutions don’t rely exclusively on OCR. At Jumio, we combine OCR with AI, machine learning, computer vision and even human review to address the shortcomings of OCR.
While it may sound self-serving, a better long-term solution is to partner with experienced integrated software solutions who have accrued the experience to refine the processes and the technologies that generally result in much higher verification accuracy and speed.