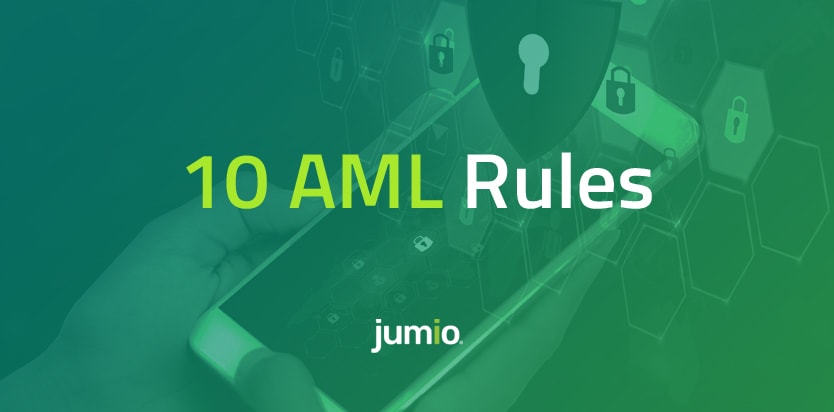
Money laundering schemes are difficult to detect. Money launderers use businesses like fintechs, banks, insurance companies, cryptocurrency dealers, gaming platforms, casinos and other financial services institutions to make their money look legitimate.
The goal of anti-money laundering programs is to find these abnormal patterns in the sea of financial transaction data generated every day within financial accounts. By implementing regulations outlined by AML laws such as the Bank Secrecy Act (BSA) and the USA Patriot Act, financial institutions and related service providers can help regulators and federal law enforcement agencies identify and prevent money laundering.
What AML Regulations Do I Need to Consider?
When establishing an AML compliance program, firms must meet a minimum standard that is set and enforced by the federal government. If a financial institution does not meet these standards, government agencies like the U.S. Treasury Department’s Financial Crimes Enforcement Network (FinCEN), the Financial Action Task Force (FATF) and the Financial Industry Regulatory Authority (FINRA) can take enforcement action against the institution in the form of a fine and, in some cases, jail time.
Compliance teams need to be aware of the regulations that apply to their specific business type and locale. They must develop the proper internal controls, including a risk assessment and customer identification program, to meet their due diligence requirements.
Digital Trust Throughout the Customer Journey
How to Leverage the Jumio Platform from Onboarding to Ongoing Monitoring
Which AML Rules Should I Use?
Complying with money laundering laws can be a real challenge, as every business situation has different risk factors and appropriate thresholds. However, there are some basic rule structures that every compliance officer should deploy to cover the most common schemes.
Below are 10 types of universal AML rules that compliance departments should consider running against all of their transactions. These rules are intended as an entry point for anyone looking to establish a compliance program.
1. Structuring Over Time
Structuring involves splitting transactions into multiple smaller transactions to avoid reporting requirements. This rule should detect an excessive proportion of transactions just below a reporting or internal threshold. For example, if the threshold is $10,000, you might look for a pattern where transactions for the party largely fall between $9,000 and $10,000 over a 60-day period.
2. Profile Change Before a Large Transaction
This rule should identify a situation when a customer makes a profile change to personally identifiable information (PII) shortly before making a large transaction. This can indicate account takeover or potential “layering” activity to obscure the path of the funds. It can also indicate the person has purchased inactive accounts and is updating them right before using them.
3. Suspicious User Spend Behavior
Similar to the previous rule, this rule helps to identify account takeover or externally influenced activity. It should identify transactions that deviate from the party’s standard spend behavior. It should also look for behavior that is outside the norms for the party’s financial profile, including their occupation, income level, marital status and education level. These thresholds must be set carefully to avoid excessive false positives.
4. Increase in Transaction Volume or Value
This rule should identify parties with abnormally high pay-out transaction volumes or a significant increase in the value of a party’s outgoing transactions compared to their recent average. A rule like this is appropriate for a peer-to-peer payment network with the capability to withdraw funds to an external account. The rule should filter out parties that have existed for a short amount of time, parties with a low balance, and low outgoing transaction value over the relevant time window.
5. Circulation of Funds
Circulation of funds occurs when parties pay themselves (self-transfer) through different accounts. For example, this rule could look for situations where 1) the party deposits casino checks, followed by 2) the purchase of bank drafts that are ultimately used at one or more casinos, and then 3) casino checks—whose memo indicates that the funds are not the result of casino winnings—are deposited back into the account. The rule should also look for transfers between parties with the same IP address.
6. Excessive Flow-through Activity
This rule should identify parties where the total value of credits is similar to the total value of debits over a short time frame. A rule like this is appropriate for a service that generally offers collection of funds where you would not expect to see comparable spend activity (for example, a marketplace for goods and services).
7. Low Number of Buyers
On platforms where you generally see many buyers (senders) interacting with a single seller (recipient), the rule should identify merchants that only receive payments from a small number of buyers. This can help identify collusion and circulation of funds. This rule should only fire for accounts older than a set threshold to validate low diversity over time and permit some time for merchants to ramp up their interaction.
8. Low Communication Between Buyers and Sellers
On platforms that track the frequency of communication between buyers and sellers on the service, this rule should identify merchants with high earnings but very few sent messages, which could indicate collusion or money laundering rather than conventional commercial activity. This rule should trigger based on an adjustable percentage threshold of messages sent per monetary unit earned. It should also identify merchants with a high percentage of their activity coming from new accounts, which is a potential red flag for money laundering or conventional fraud.
9. High-Risk Jurisdictions
This rule should use geographic-based risk indicators for countries and regions where money laundering is often found. Some examples of risk categories include high banking secrecy, high financial crime, high drug trafficking and known tax havens. It’s important to keep this rule updated based on the latest intelligence. For example, in June of 2021, the FATF updated its list of jurisdictions under monitoring to include Haiti, Malta, the Philippines and South Sudan, and it removed Ghana from the list.
10. Anonymizing the Source of Funds
This rule should look for situations where the party sends funds into decentralized exchanges (DEXes) and then extracts it later, which can be used to anonymize the source of funds. It should also identify when the party converts their currency into a gaming token and then withdraws it. Both of these activities raise the risk of money laundering.
While these AML rules cover the basics, they are just a few of the rules you need to fully protect your business and the financial system to help stop crimes such as human trafficking and terrorist financing. You also need to make sure your rules evolve over time and are tailored to your business so you don’t burden your compliance team with false positives.
Of course, the best way to stop money laundering is to prevent money launderers from ever getting onto your platform in the first place. Visit our website to learn how Jumio helps companies meet AML compliance mandates.
Updated March 24, 2023