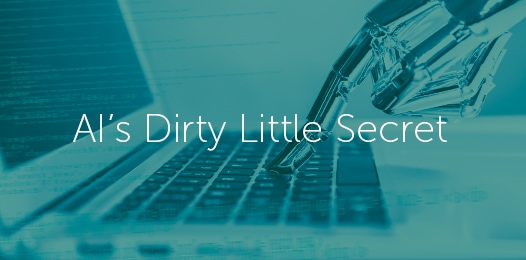
The promise of Augmented Intelligence is undeniable.
At this year’s Google I/O developer conference, Google demoed a very natural-sounding Google Assistant making an appointment over the phone — a feature it calls Google Duplex. This wasn’t just auto-filling an online form — this was a phone call between Google’s AI Assistant and a human working at a salon. It’s amazing.
More recently, a Stanford-led study demonstrated that in a matter of seconds, a new algorithm read chest X-rays for 14 pathologies, performing as well as radiologists in most cases. A new artificial intelligence algorithm can reliably screen chest X-rays for more than a dozen types of disease, and it does so in less time than it takes to read this sentence. The algorithm is the first to simultaneously evaluate X-rays for a multitude of possible maladies and return results that are consistent with the readings of radiologists, the study says.
So, surely Augmented Intelligence can be applied to stopping fraud and reliably verifying someone’s online identification, right? The answer is mostly yes. AI and machine learning are making quantum improvements in fraud detection, but there are still limits.
At Jumio, we’ve spent the last several years developing AI models that push the boundaries that help us to reliably extract key data from government-issued IDs, find anomalies in manipulated IDs (looking for all the embedded security features), ensure the person is physically present with AI-enriched liveness detection and develop risk scores based on evolving fraud characteristics.
But Augmented Intelligence, when used in isolation, has limitations in the world of online identity verification. Let’s review a few of them:
- The Need for Big Data. Consider the words of Joel Tetreault, director of research at Grammarly: “But a dirty little secret about industrial-strength AI is that many of these systems are trained and evaluated on datasets created and labeled by thousands of human raters.” If you’re going to be an AI model for Nepalese passports, you need to inspect a large number of Nepalese passports, both fraudulent and legitimate, to properly train your machine learning algorithms.
- The Number of ID Types & Subtypes. One of the challenges of AI is supporting the broad range of ID types and subtypes. For example, a California driver’s license can look different depending on a number of factors including the type of vehicle, whether the vehicle is used for commercial or non-commercial purposes and the age of the driver (California now issues vertically oriented driver’s licenses for provisional drivers and anyone under 21 years of age). Each one of these subtypes requires a different ID template, each with its own unique security features and the text (such as name, address and date of birth) is in physically different locations on the ID. At Jumio, we support over 3,500 ID types and subtypes — and that imposes challenges on our AI models since they need to address all the nuances of each ID subtype which are continually changing.
- Blurriness, Bad Lighting and Glare. Unlike Stanford’s X-rays, the picture quality of a government-issued ID can vary wildly. Most modern smartphones come factory-loaded with high-quality cameras that can auto-focus. But, this is not true of all mobile phones. Plus, some pictures of IDs are shot in bad light or with glare. Most AI algorithms do not perform well in these less-than-ideal circumstances.
- The Challenge of Omnichannel. At Jumio, we encourage our customers to offer their downstream customers a choice of channels for capturing a picture of their ID. Many will naturally use their smartphones when they’re on the go, but other folks prefer to use the webcam in their desktop or laptop. But, the choice of channel actually adds complexity for AI models. For example, the quality of images captured by webcams is notoriously bad because there is no auto-focus. That’s why some companies only allow photos to be captured via a mobile SDK, but this will limit the reach of your potential customers.
- The Selfie Requirement. It’s not only the ID that needs to get verified, it’s the corroborating selfie that many online companies are now requiring to ensure that the picture in the selfie matches the picture in the government-issued ID. Assuming there is only one person in the selfie, the person is facing the camera, and the person hasn’t changed too much since they took the picture in their ID, the face-matching process is fairly trivial, but I’m describing ideal circumstances. As you stray from the ideal, the challenges for AI become more acute.
- Liveness Detection. We’ve started to see a rise in spoofing attacks by fraudsters to acquire someone else’s privileges or access rights. They do this by using a photo, video or a different substitute for an authorized person’s face. Machine learning models use statistical analysis of historical data to predict potential fraud, but these types of spoofing attacks can stump an AI algorithm.
Augmented Intelligence to the Rescue
Given these current limitations of AI, there’s a clear need for humans to ensure that the right verification decision is made online.
That’s where augmented intelligence comes in.
Augmented Intelligence fuses technology with human expertise. The role of AI may become greater in time, but the state of technology still requires a human element — if for nothing else than to tag and train our algorithms and make them iteratively smarter.
We think of augmented intelligence as Jarvis, the AI built into Iron Man’s armor — it’s the technology that gives Tony Stark super powers. At Jumio, we’re leveraging the power and insights from 150 million verifications to help develop better AI models and leveraging human AI agents to not only verify suspect IDs and selfies, but to also inform our models when they’re making the correct decisions and when they’re missing the mark.
While big data is important, you also need to have the data intelligently tagged. At Jumio, we’ve hired qualified verification experts and AI trainers to tag ID documents — both good and rejected images — and to help train our ML algorithms. Image tagging is based on the answering the following questions:
- Was the image scuffed?
- Was the ID hole punched?
- Which country was the ID from?
- Was there glare?
- Was part of the picture obscured by a thumb?
By tagging tens of thousands of IDs in this manner, the algorithms that feed machine learning get smarter, faster and learn how to recognize these patterns automatically. We’re also using our AI models to flag areas on the idea that require closer inspection — these could be areas of high glare or suspect fonts that may not match the ID template. Like Jarvis, Jumio’s AI models are helping our verification experts focus their attention to help make the best verification speed, while also speeding up the verification process.
But, in order to capitalize on augmented intelligence, you need to ensure that your chosen identity verification solution provider has these ingredients in place:
Big Data. For identity companies, this means capturing government-issued IDs in large volumes to train their algorithms to spot patterns and better detect when an ID has been manipulated or altered in any way. But, this is AI’s dirty little secret as most companies don’t have sufficient data to create AI models that are highly predictive.
Human Review. To better inform the algorithms, there needs to be a continuous feedback loop where every ID (and face matching image pair) is labeled as pass or fail. When only a small fraction of transactions is reviewed by humans (as is often the case with automated solutions), this limits the ability of deep learning.
Data Scientists. Increasingly, data scientists are in high demand to help build deep learning models. Make sure your identity verification vendor has made these personnel investments to exploit the potential of artificial intelligence.
Years of Experience. Companies that are new to the identity verification space are disadvantaged in some pretty material ways when it comes to ML and deep learning. Usually, they have not amassed much data to inform their algorithms. But, just as importantly, their verification experts often don’t have the experience to know how to tag legitimate and fraudulent ID documents and are often only reviewing a handful of online verifications. Leading companies that have long relied on human review are in a much better position to recognize fraudulent IDs and face matches because of their experience and training.
Strict Privacy Compliance. Jumio treats data privacy compliance as a mandatory requirement of any solution released to the market. This includes being fully GDPR compliant and operating and conforming to strict PCI-DSS data privacy requirements. This impacts how we develop our AI algorithms and ensure our business customers and their downstream users’ privacy is always respected.
We will keep innovating and pushing the limitations of AI, but it’s becoming increasingly important for our customers to understand that AI does have limitations (currently) and that those limitations can impact the quality of the online identity verification process and fraud detection.